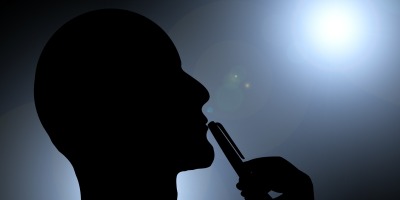
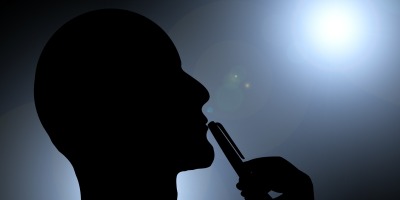
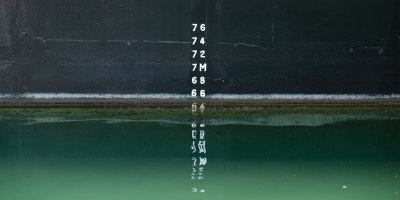
Evaluation, Intelligence, Reasoning, Tradecraft
What might an alternative to the ODNI Rating Scale be like?
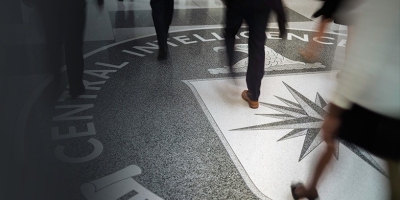
Evaluation, Expertise, Intelligence, Tradecraft
The ODNI Rating Scale – Issues Abound
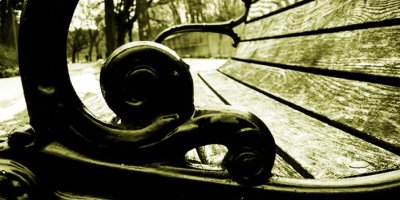
A revolution in spycraft?
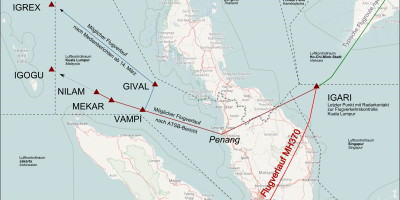
Citizen Intelligence, Crowdsourcing, Intelligence
Challenges in getting a citizen intelligence marketplace up and running
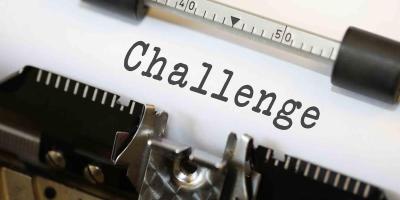
Citizen Intelligence, Crowdsourcing, Intelligence
A challenge model for citizen intelligence
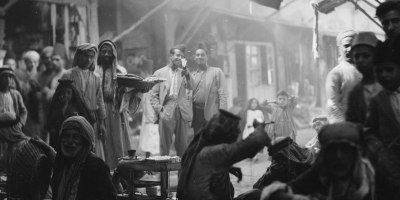
Citizen Intelligence, Intelligence
Citizen intelligence lacks a marketplace
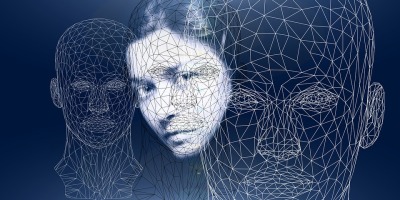
Intelligence, Prediction, Teams
Three new papers relevant to improving analysis
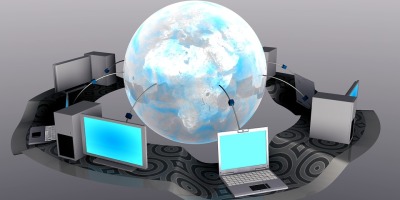
Citizen Intelligence, Intelligence
Citizen Intelligence is fast becoming a real thing
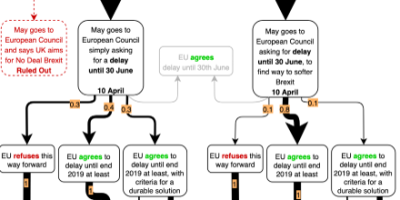
Forecasting, Intelligence, Visualization